Unveiling Patterns: The Art of Data Analysis
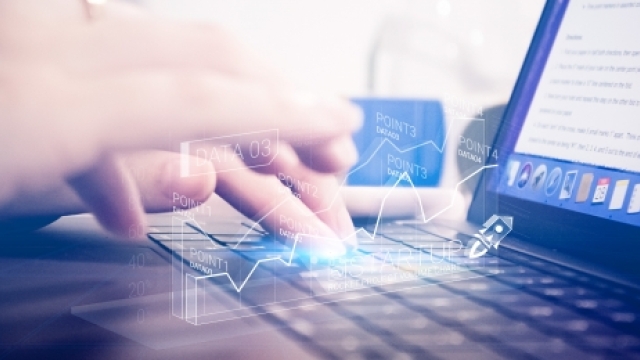
As we immerse ourselves in a world overflowing with information, the ability to extract meaningful insights from data has become a fundamental skill across various industries. Data analysis serves as a powerful tool, enabling individuals and organizations to navigate through the vast sea of data, uncovering hidden patterns and valuable knowledge. Whether it be making informed business decisions, predicting future trends, or understanding behavioral patterns, the art of data analysis underpins much of the progress and innovation we witness today. Through a systematic approach of collecting, cleaning, processing, and interpreting data, analysts transform raw information into actionable intelligence, driving efficiency and success in their endeavors.
Methods of Data Analysis
In the field of data analysis, there are various methods used to extract meaningful insights from datasets. One common approach is descriptive analysis, which involves summarizing the main characteristics of a dataset. This method helps in understanding the basic features of the data and providing simple summaries about the samples.
Another essential method is inferential analysis, where the goal is to draw conclusions that extend beyond the immediate data alone. By using statistical techniques, inferential analysis allows data analysts to make inferences or predictions about a larger population based on a smaller sample of data. This method is crucial in making decisions and predictions in various fields such as business, healthcare, and social sciences.
Furthermore, exploratory data analysis is a method that focuses on exploring the data to understand its structure and to extract insights that can lead to more focused investigations. This approach involves techniques like data visualization, clustering, and dimensionality reduction. Exploratory data analysis plays a vital role in the initial stages of data analysis, helping analysts identify patterns and relationships within the data before performing more in-depth analyses.
Importance of Data Visualization
Data visualization is a crucial component of effective data analysis. Through visual representations such as graphs, charts, and maps, complex datasets can be transformed into clear and digestible insights. Visualization allows for patterns, trends, and outliers to be easily identified, enabling data analysts to draw meaningful conclusions and make informed decisions.
By presenting data in visual formats, data visualization enhances communication and understanding among stakeholders. Visualizations can simplify complex information, making it more accessible to individuals with varying levels of data literacy. This fosters collaboration and enables teams to work together more effectively towards common goals, leveraging the power of visual storytelling to convey key messages.
Furthermore, data visualization can reveal hidden relationships and correlations that may not be immediately apparent from raw data. Through interactive dashboards and dynamic visualizations, analysts can explore data from different perspectives, uncovering valuable insights that drive business strategies and innovation. Visualization not only enhances data analysis processes but also enables organizations to harness the full potential of their data assets.
Challenges in Data Interpretation
Data interpretation can present several challenges, particularly in the context of vast datasets and complex relationships. One common issue is the presence of outliers, which can skew the analysis and lead to misleading conclusions. Identifying and appropriately handling outliers is essential in ensuring the accuracy of the data interpretation process.
Another challenge in data interpretation lies in dealing with missing or incomplete data. Gaps in the dataset can hinder the analysis and compromise the validity of the results. Imputation techniques and careful consideration of the implications of missing data are crucial to overcoming this challenge and ensuring a robust interpretation.
Moreover, the interpretation of data can be challenging when there is ambiguity or conflicting information. In such cases, it is important to delve deeper into the data, consider different perspectives, and possibly seek additional sources or expert opinions to clarify the findings. Navigating through ambiguous data requires critical thinking and a keen eye for detail to arrive at meaningful interpretations.